Written by: Vasiliki Maria Loukaki
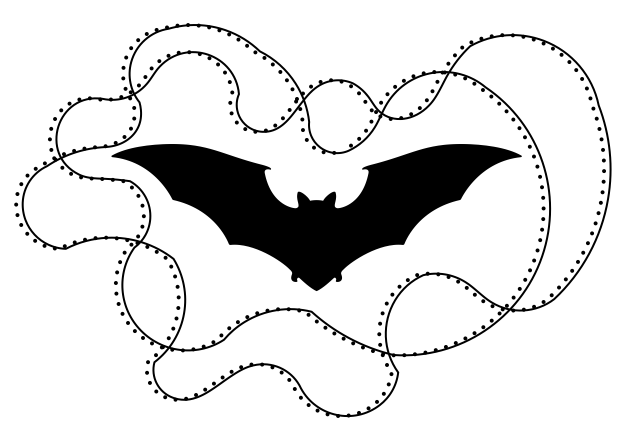
From predator to prey, carnivore to herbivore, vertebrate to invertebrate, the lives of animals appear rather distinct from one another. Yet, upon closer inspection, a series of common denominators is revealed, like a long piece of thread tying them all together. Navigation is inarguably one of these denominators, underlying their ability to forage, hunt, migrate, nest, breed, colonise, and much more. The neural underpinnings of navigation have consistently been the focus of neuroscience research ever since the momentous discovery of place cells by O’Keefe and Dostrovsky (1971). Subsequent research has revealed how humans and other animals are able to encode a mental representation of the physical world, in order to position themselves in relation to their spatial surroundings (O’Keefe & Nadel, 1978; Taube et al., 1990; Hafting et al., 2005). However, theories vary when explaining how this mental representation – commonly referred to as a cognitive map – underlies a crucial constituent of navigation; the ability to reach a spatial goal.
In their study of Egyptian fruit bats, Sarel et al. (2017) take a significant step towards deciphering the computation of goal-directed navigation by presenting the first neural evidence that supports a vector-based approach to encoding spatial goals in mammals.
Historical Context
The hippocampus and adjoining brain regions have been repeatedly acknowledged as an instrumental influence on navigation skills. This is primarily supported by evidence for the existence of place cells, grid cells, head-direction cells, and others that enable the construction of a cognitive map that has been speculated to underlie one’s navigation through the physical world (Moser et al., 2008; Spiers & Barry, 2015; Behrens et al., 2018). Although these cells are efficient at encoding the location and orientation of the individual in space, they do so irrespectively of spatial goals. This marks a crucial gap in navigation research, especially when considering that the ability to reach a spatial goal is the entire purpose of navigation in the first place.
Certain computational models have attempted to utilise properties of the aforementioned cells in order to explain how they could give rise to goal-directed navigation (Burgess, 2008). For example, theories have posed that goal-directed navigation involves planning mental routes by activating a temporal sequence of place cells that connects the location of the individual to the location of their goal (Johnson & Redish, 2007). In fact, Burgess and O’Keefe (1996) proposed one of the earliest versions of this type of model (i.e., Simple Model of Navigation). Nevertheless, no clear neural evidence has been put forward to substantiate such models.
Other computational models have postulated the existence of a vectorial representation of spatial goals. Geometrically, a vector is represented as a line segment with a specific direction and length. Therefore, a vectorial representation of spatial goals would suggest the existence of neurons that encode the direction and distance of the individual in relation to the goal. Once again, Burgess and O’Keefe (1996) proposed one of the earliest versions of this type of model (i.e., Population Vector Model of Navigation). However, apart from behavioural studies that have proposed vector-based navigation as the mechanism underlying path integration in different animals, including ants (Müller & Wehner, 1988), hamsters (Etienne et al., 1998), and humans (Foo et al., 2005), no clear neural evidence has been put forward to substantiate such models.
This was until Sarel et al. (2017) published their paper on Egyptian fruit bats, presenting the first neural evidence of hippocampal goal-vector cells (GVCs) that could constitute the building blocks of the vectorial representation of spatial goals that had been theoretically contemplated for over two decades.
Experimental Design
Sarel et al. (2017) recorded the activity of 309 hippocampal neurons from the CA1 subfield of three Egyptian fruit bats. These model animals have been widely used in navigation research, particularly due to their heads being sufficiently large to carry the wireless electrophysiology device that uses implanted electrodes to collect single-neuron recordings, while simultaneously allowing the test subjects to fly around unrestrained. Notably, these researchers have worked with Egyptian fruit bats before, having been able to discover place cells, grid cells and head-direction cells within their hippocampal formation (Rubin et al., 2014; Finkelstein et al., 2015; Geva-Sagiv et al., 2015). According to this particular experimental setup, the test subjects were able to fly around a room with a singular, standalone platform. This platform was stocked with food, conditioning the test subjects to compute it as their spatial goal.
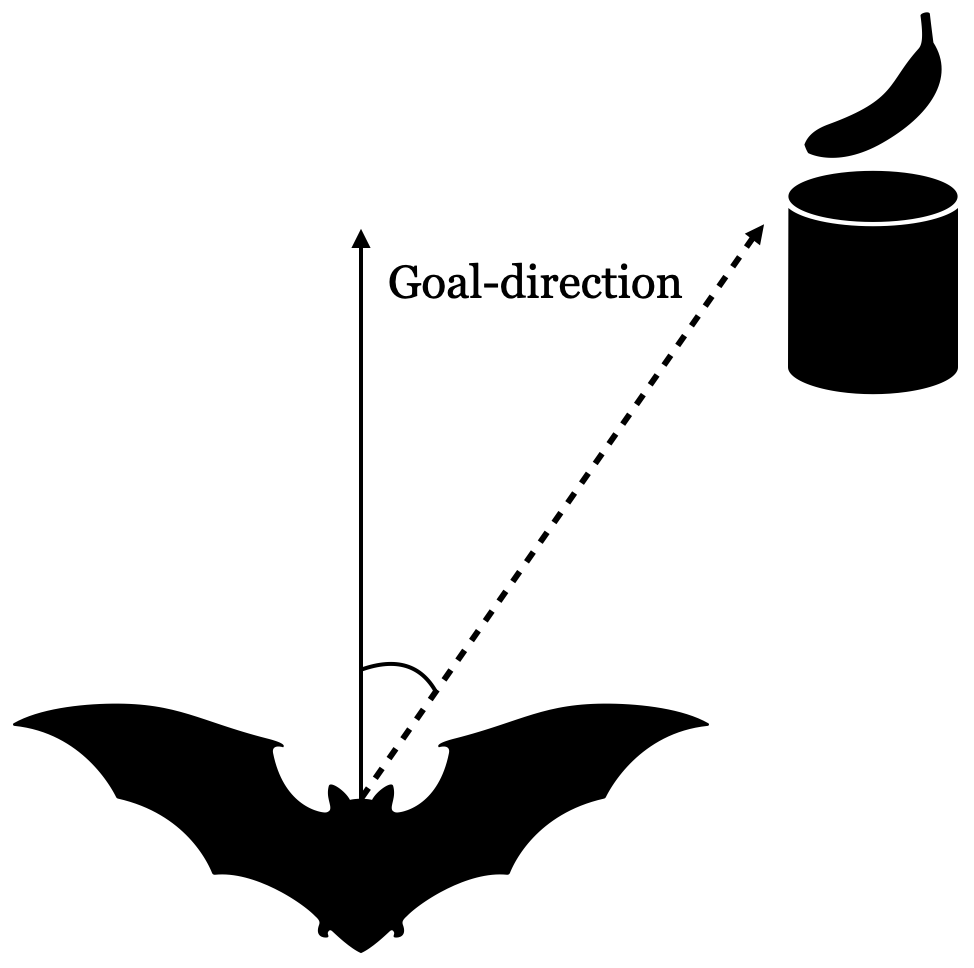
Principal Results
Sarel et al. (2017) discovered hippocampal neurons that exhibited goal-direction tuning and goal-distance tuning. More specifically, they discovered that 19% of the cells they recorded from were goal-direction cells (i.e., selectively increasing their activity whenever the bat was facing at a specific angle away from the spatial goal) and 16% of the cells were goal-distance cells (i.e., selectively increasing their activity whenever the bat was located at a specific distance away from the spatial goal). Additionally, they discovered that a significant portion of those cells exhibited both goal-direction tuning and goal-distance tuning. These are GVCs and they selectively increase their activity both in response to when the individual is located at a specific angle away from the spatial goal and a specific distance away from the spatial goal. The researchers interpreted this finding as neural evidence in support of the vectorial representation of spatial goals. The fact that singular neurons are able to encode the characteristics of a vector in relation to a spatial goal entails that a population of said neurons would theoretically be able to combine their input and allow the individual to compute a continuously updated representation of its location and orientation in relation to the goal. Thus, making it possible for the individual to decode its progress by gradually minimising the angle and distance between itself and the goal, before ultimately reaching said goal.
Historical Contribution
Although GVCs have not since been discovered in other model animals (Whittington et al., 2022) they have significantly contributed to an upsurge in the discovery of vector-based models of navigation. An example of this is the discovery of object-vector cells in the entorhinal cortex of mice that seem to function similarly to GVCs with the exception of being tuned to salient objects rather than specific goals (Høydal et al., 2019). Moreover, GVCs have instigated the development of computational models that combine the two aforementioned theoretical approaches, illustrating the idea that vectorial computations are being used in tandem with place cell (Ormond & O’Keefe, 2022) and grid cell (Banino et al., 2018) activation to mediate goal-directed navigation.
Conclusion
Overall, the discovery of GVCs has undoubtedly broadened our understanding of goal-directed, vector-based navigation. Given that these aspects of navigation are somewhat underrepresented in academic literature – yet underlie the very functionality of navigation skills – the contributions of Sarel et al. (2017) are a landmark of advancement in the field. That being said, the fact that equivalent cells have not been discovered in other animal models is rather disappointing. Although, recent findings have revealed the existence of cells with similar coding properties in humans (Kunz et al., 2021). Further research should attempt to identify similar cells in different model animals to establish their interspecies validity as practical mediators of navigation.
A modern reconceptualisation of spatial coding has suggested that the same cells used to construct a mental representation of physical space to underlie navigation (e.g., place cells, grid cells etc.) are also being used to construct a mental representation of an “abstract” space to underlie conceptual skills (Behrens et al., 2018; Whittington et al., 2022). Perhaps, just like GVCs are being used to compute one’s approach of a spatial goal in physical space, they may also be useful for computing one’s approach of a social goal in “abstract” space – where the measures of direction and distance are more figurative.
References:
Banino, A., Barry, C., Uria, B., Blundell, C., Lillicrap, T., Mirowski, P., Pritzel, A., Chadwick, M. J., Degris, T., Modayil, J., Wayne, G., Soyer, H., Viola, F., Zhang, B., Goroshin, R., Rabinowitz, N., Pascanu, R., Beattie, C., Petersen, S., & Sadik, A. (2018). Vector-based navigation using grid-like representations in artificial agents. Nature, 557(7705), 429–433. https://doi.org/10.1038/s41586-018-0102-6
Behrens, T. E. J., Muller, T. H., Whittington, J. C. R., Mark, S., Baram, A. B., Stachenfeld, K. L., & Kurth-Nelson, Z. (2018). What Is a Cognitive Map? Organizing Knowledge for Flexible Behavior. Neuron, 100(2), 490–509. https://doi.org/10.1016/j.neuron.2018.10.002
Burgess, N. (2008). Spatial Cognition and the Brain. Annals of the New York Academy of Sciences, 1124(1), 77–97. https://doi.org/10.1196/annals.1440.002
Burgess, N., & O’Keefe, J. (1996). Neuronal computations underlying the firing of place cells and their role in navigation. Hippocampus, 6(6), 749–762. https://doi.org/10.1002/(SICI)1098-1063(1996)6:6<749::AID-HIPO16>3.0.CO;2-0
Etienne, A. S., Maurer, R., Berlie, J., Reverdin, B., Rowe, T., Georgakopoulos, J., & Séguinot, V. (1998). Navigation through vector addition. Nature, 396(6707), 161–164. https://doi.org/10.1038/24151
Finkelstein, A., Derdikman, D., Rubin, A., Foerster, J. N., Las, L., & Ulanovsky, N. (2014). Three-dimensional head-direction coding in the bat brain. Nature, 517(7533), 159–164. https://doi.org/10.1038/nature14031
Foo, P., Warren, W. H., Duchon, A., & Tarr, M. J. (2005). Do Humans Integrate Routes Into a Cognitive Map? Map- Versus Landmark-Based Navigation of Novel Shortcuts. Journal of Experimental Psychology: Learning, Memory, and Cognition, 31(2), 195–215. https://doi.org/10.1037/0278-7393.31.2.195
Geva-Sagiv, M., Las, L., Yovel, Y., & Ulanovsky, N. (2015). Spatial cognition in bats and rats: from sensory acquisition to multiscale maps and navigation. Nature Reviews Neuroscience, 16(2), 94–108. https://doi.org/10.1038/nrn3888
Hafting, T., Fyhn, M., Molden, S., Moser, M.-B., & Moser, E. I. (2005). Microstructure of a spatial map in the entorhinal cortex. Nature, 436(7052), 801–806. https://doi.org/10.1038/nature03721
Høydal, Ø. A., Skytøen, E. R., Andersson, S. O., Moser, M.-B., & Moser, E. I. (2019). Object-vector coding in the medial entorhinal cortex. Nature, 568(7752), 400–404. https://doi.org/10.1038/s41586-019-1077-7
Johnson, A., & Redish, A. D. (2007). Neural Ensembles in CA3 Transiently Encode Paths Forward of the Animal at a Decision Point. Journal of Neuroscience, 27(45), 12176–12189. https://doi.org/10.1523/jneurosci.3761-07.2007
Kunz, L., Brandt, A., Reinacher, P. C., Staresina, B. P., Reifenstein, E. T., Weidemann, C. T., Herweg, N. A., Patel, A., Tsitsiklis, M., Kempter, R., Kahana, M. J., Schulze-Bonhage, A., & Jacobs, J. (2021). A neural code for egocentric spatial maps in the human medial temporal lobe. Neuron, 109(17), 2781-2796.e10. https://doi.org/10.1016/j.neuron.2021.06.019
Moser, E. I., Kropff, E., & Moser, M.-B. (2008). Place Cells, Grid Cells, and the Brain’s Spatial Representation System. Annual Review of Neuroscience, 31(1), 69–89. https://doi.org/10.1146/annurev.neuro.31.061307.090723
Muller, M., & Wehner, R. (1988). Path integration in desert ants, Cataglyphis fortis. Proceedings of the National Academy of Sciences, 85(14), 5287–5290. https://doi.org/10.1073/pnas.85.14.5287
O’Keefe, J., & Dostrovsky, J. (1971). The hippocampus as a spatial map. Preliminary evidence from unit activity in the freely-moving rat. Brain Research, 34(1), 171–175.
O’Keefe, J., & Nadel, L. (1978). The hippocampus as a cognitive map. Oxford University Press.
Ormond, J., & O’Keefe, J. (2022). Hippocampal place cells have goal-oriented vector fields during navigation. Nature, 607(7920), 741–746. https://doi.org/10.1038/s41586-022-04913-9
Rubin, A., Yartsev, M. M., & Ulanovsky, N. (2014). Encoding of Head Direction by Hippocampal Place Cells in Bats. The Journal of Neuroscience, 34(3), 1067–1080. https://doi.org/10.1523/jneurosci.5393-12.2014
Sarel, A., Finkelstein, A., Las, L., & Ulanovsky, N. (2017). Vectorial representation of spatial goals in the hippocampus of bats. Science, 355(6321), 176–180. https://doi.org/10.1126/science.aak9589
Spiers, H. J., & Barry, C. (2015). Neural systems supporting navigation. Current Opinion in Behavioral Sciences, 1, 47–55. https://doi.org/10.1016/j.cobeha.2014.08.005
Taube, J., Muller, R., & Ranck, J. (1990). Head-direction cells recorded from the postsubiculum in freely moving rats. I. Description and quantitative analysis. The Journal of Neuroscience, 10(2), 420–435. https://doi.org/10.1523/jneurosci.10-02-00420.1990
Whittington, J. C. R., McCaffary, D., Bakermans, J. J. W., & Behrens, T. E. J. (2022). How to build a cognitive map. Nature Neuroscience, 25(10), 1257–1272. https://doi.org/10.1038/s41593-022-01153-y
Comments